GSI Technology: More Than A Meme And A Viable Alternative To Nvidia
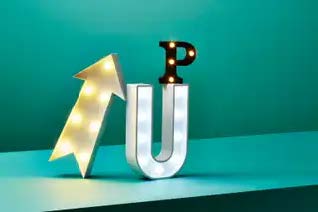
Summary
- GSI Technology reported revenues of $5.4M in recently reported Q4 2022, with R&D expenses of $5.0M as it reinvests most of its revenues to enhance its product line.
- GSI Technology said its Gemini-I APU outperforms other types of processors including those of Nvidia, Intel, and Graphcore.
- GSI Technology is expanding work on vector search engine applications for Gemini-I, a fundamental part of ChatGPT architecture.
- The Gemini-II APU, in production within the next 12 months, has more than four times the processing power and eight times of memory density compared to Gemini-I.
- This idea was discussed in more depth with members of my private investing community, Semiconductor Deep Dive.
Strong Technology and Advanced Processor in the Wings
GSI Technology (NASDAQ: GSIT) is the developer of the Gemini Associative Processing Unit (“APU”) for Artificial Intelligence (“AI”) and high-performance parallel computing solutions for the networking, telecommunications, and military markets.
Its APUs, in comparison to Nvidia’s (NVDA) GPUs (Graphical Processing Units), offer 93% reduced power consumption when running AI search applications in datacenters while enabling customers to improve their environmental and sustainability efforts. This performance has been achieved with its Gemini-I APU.
On its recent earnings call of May 17, 2023, GSIT reported that its Gemini-II APU has more than four times the processing power and eight times of memory density compared to Gemini-I.
Gemini-II is built with TSMC’s 16nm process. The chip contains 6 Megabyte of associative memory connected to 100 Megabyte distributed SRAM with 45 Megabyte per second bandwidth or 15 times the memory bandwidth of the state-of-the-art parallel processor for AI.
GSIT is on track to complete the tape-out for Gemini-II this summer and evaluate the first silicon chip by the end of 2023.
Importantly in the ChatGPT generative AI space, Lee-Lean Shu – Chairman, President and CEO
reported:
“Looking ahead on our roadmap, we will build upon the work we are doing today in future APU versions to address large language model or LLM for natural language processing. Vector search engines are a fundamental part of ChatGPT architecture and essentially function as the memory for ChatGPT. Large language models use deep neural networks, such as transformers, to learn billions or trillions of words and produce text. This is another reason that vector search is an appropriate focus application with the APU.“
More than a Meme Stock
A “meme” stock is a stock that may see dramatic increases in price, mostly fueled by social media discussions on Reddit and Twitter. That can be said of GSIT. Indeed, prior to its earnings call on May 16, GSIT share price increased more than 100% on May 15, the day before its earnings call.
GSI reported a net loss of $4 million, or $0.16 per diluted share, on net revenues of $5.4 million for the fourth quarter of fiscal 2023, compared to a net loss of $3 million, or $0.12 per diluted share, on net revenues of $8.7 million for the fourth quarter of fiscal 2022 and a net loss of $4.8 million, or $0.20 per diluted share, on net revenues of $6.4 million for the third quarter of fiscal 2023.
Importantly for investors, research and development (R&D) expenses were $5 million, compared to $6.5 million in the prior-year period and $5.5 million in the prior quarter. R&D was essentially equal to revenues, meaning management is willing to forego profits for growth in the company.
Nvidia is Not the Only AI Chip Solution
I’ve written several Seeking Alpha articles about Nvidia and its AI solutions. Importantly, in my Sept. 22, 2020, article entitled “Nvidia Needs ARM To Revitalize Its AI Goals” I noted in the bullets that:
- Nvidia has been the dominant leader in GPUs for AI datacenter training but will lose market share to competing chip technologies.
- Nvidia faces even stronger competition from other chip technologies at datacenter AI inference.
- As with datacenter, Nvidia faces strong competition from FPGA and ASIC accelerator chips in edge server.
I noted that, and as background:
“There are two components of AI at the data center. Training reevaluates or adjusts the layers of the neural network based on the results. Training a leading AIgorithm can require a month of enormous computational power. Inference applies knowledge from a trained neural network model and uses it to infer a result. AI chips are mostly used to apply trained AI algorithms to real-world data inputs; this is often called ‘inference.’“
In other words, Nvidia’s GPU chips dominated AI at the Training, but at the Inference phase, other processor architectures have benefits. Nvidia’s CUDA has had a monopoly in machine learning because it was the only game in town. That dominance may be changing as more organizations move to OpenAI’s Triton and PyTorch 2.0. Both Triton and PyTorch 2.0 are faster and easier to use than CUDA. PyTorch 2.0 is an open source machine learning library released in March. OpenAI, best known for its generative AI like ChatGPT, with its Triton software, facilitate straining of large-scale machine learning models on parallel computing hardware.
Overall, Chart 1 shows the AI Datacenter chip forecast by chip type, according to our report entitled “Hot ICs: A Market Analysis of Artificial Intelligence (AI), 5G, Automotive, and Memory Chips,” illustrating the erosion of Nvidia’s share by other chip types, namely ASICs (application specific integrated circuits) and FPGAs (field programmable gate arrays).
CHART 1
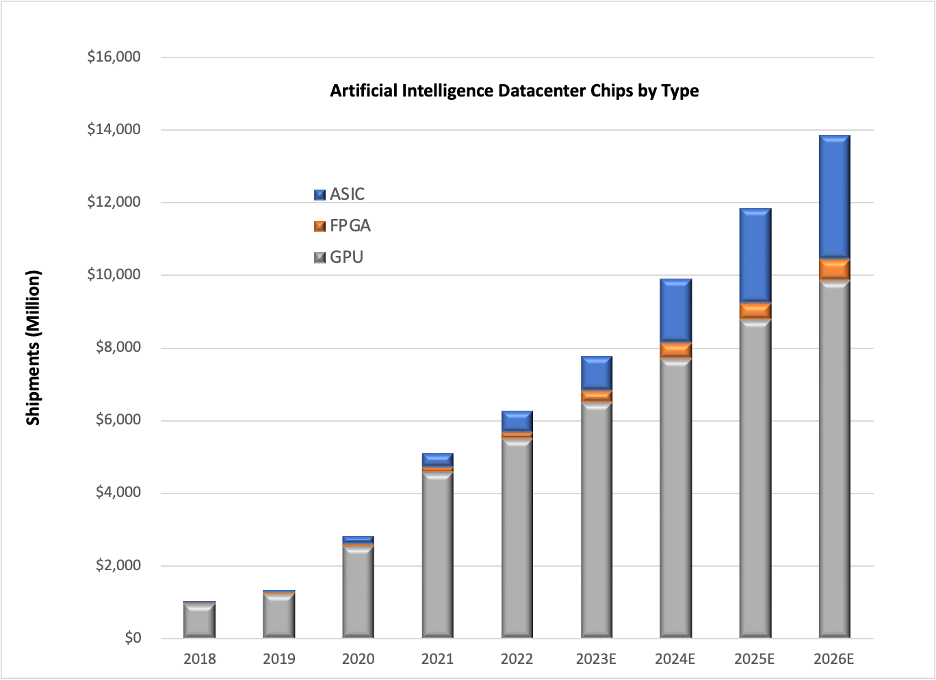
Enter GSI's APU
The IP behind the APU (associative processing unit) architecture was acquired by GSI in 2015 with the acquisition of Israel-based MikaMonu, then combined with GSI’s SRAM technology to enable a new, additional direction for the company.
The Gemini APU combines SRAM and two million bit-processors for in-memory computing functions. SRAM – short for Static Random Access Memory – is faster and more expensive than DRAM.
The GSI interweaves 1-bit processing units with the read-modify-write lines of SRAM in its Gemini chip. All these processors work in parallel.
As shown in Table 1, Gemini-I outperforms other types of processors. A Gemini-I chip can perform two million x 1-bit operations per 600MHz clock cycle with a 39 TB/sec memory bandwidth, whereas an Intel (INTC) Xeon 8280 can do 28 x 2 x 512 bits at 2.7GHz with a 1TB/sec memory bandwidth.
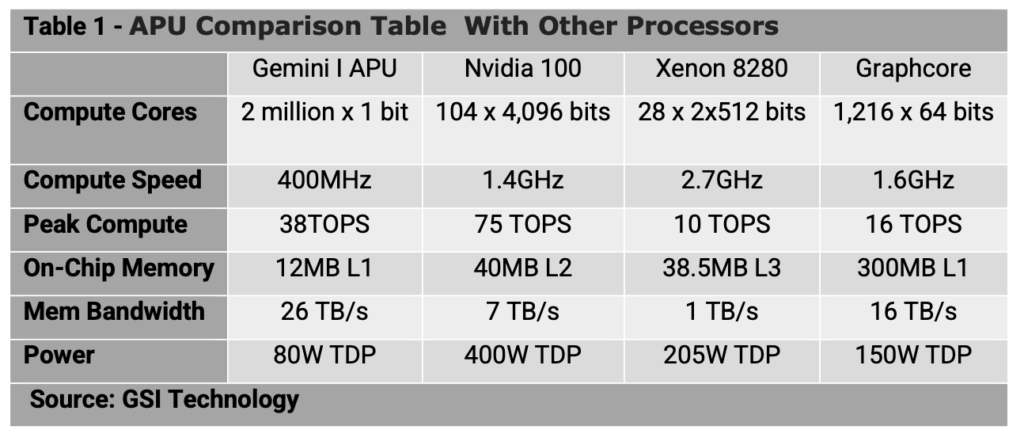
For comparison, a Nvidia A100 GPU server can complete 104 x 4,096 bits per 1.4GHz clock cycle, providing a 7TB/sec memory bandwidth. Furthermore, the data rate from the GPU’s large L2 cache is 7TB/s, still only 27% of Gemini’s. For rapid data searches, the GPU will bottleneck on this limited bandwidth. The Nvidia chip requires even more power than the biggest Xeon chip.
Graphcore has developed a chip that has even more processors: 1,216 cores. Like the GPU, however, this chip is optimized for neural networks and only achieves its peak rate of 250 TOPS for matrix multiplication. For general-purpose calculations, the cores can reach 16 TOPS.
Gemini-II is built with TSMC’s 16nm process. The chip contains 6 Megabyte of associative memory connected to 100 Megabyte distributed SRAM with 45 Terabyte per second bandwidth or 15 times the memory bandwidth of the state-of-the-art parallel processor for AI. This is more than four times the processing power and eight times of memory density compared to Gemini-I.
GSIT is on track to complete the tape-out for Gemini-II this summer and evaluate the first silicon chip by the end of 2023. The company aims to bring this solution to market in the second half of 2024.
Chart 2 illustrates that traditional processors (left) struggle with large datasets due to the narrow connection with the large on-chip memory. Gemini features millions of cores that can all access memory at once, allowing a much greater flow of data.
CHART 2
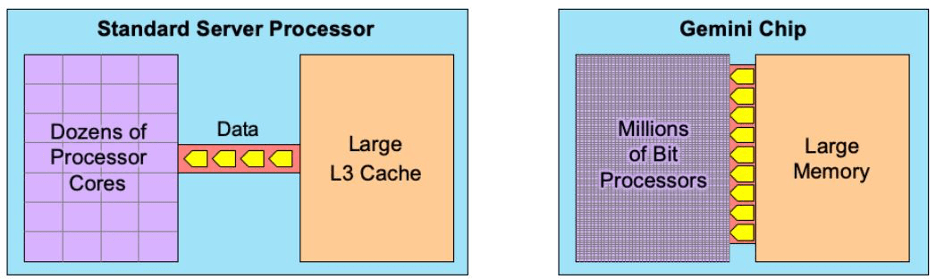
Table 1 above also shows that the power of the four chips. According to GSI Technology, power consumption is a serious problem today in the data center. According to a recent study, today’s data centers consume an estimated 198-terawatt hours or 1% of global electricity use. The amount of energy used by datacenters doubles approximately every four years, meaning that data centers have the fastest-growing carbon footprint within the IT sector.
Investor Takeaway
GSIT was established in 1995, so it’s not a startup, but its APU technology was acquired more recently in 2015. An attribute is that the Gemini-I APU’s architecture features parallel data processing with two million-bit processors per chip. The massive in-memory processing reduces computation time from minutes to milliseconds, while significantly reducing power consumption in a scalable format. As shown in a comparison of leading AI chips, the Gemini excels compared to Intel, Nvidia, and Graphcore.
Gemini-I excels at large (billion item) database search applications like facial recognition, drug discovery, Elasticsearch, and object detection.
The upcoming introduction of the Gemini-II will enable the company to continue to gain traction in generative AI like ChatGPT. GSIT is expanding work on vector search engine applications for Gemini-I, and will build on that work in Gemini-II to address large language model or LLM for natural language processing.
Vector search engines are a fundamental part of ChatGPT architecture and essentially function as the memory for ChatGPT. Large language models use deep neural networks, such as transformers, to learn billions or trillions of words and produce text. This is another reason that vector search is an appropriate focus application with the APU.
This free article presents my analysis of this semiconductor equipment sector.